How OpenAI Grows
The need-to-know primer on the AI industry + a deep dive on OpenAI, their big picture strategy, and their tactics with ChatGPT and Microsoft.
Hi, I’m Jaryd. 👋 I write in-depth analyses on the growth of popular companies, including their early strategies, current tactics, and actionable business-building lessons we can learn from them.
Plus, every Friday I bring you summarized insights, frameworks, and ideas from the best entrepreneurs, writers, investors, product/growth experts, and operators.
Hi friends 👋
While losing a game of Monopoly Deal the other night, Julia (my fiancé and generous proof reader) said to me: “imagine what it must have been like to be alive and witness the first airplane, and then also see us launch rockets and go to space”.
My knee-jerk response was, “that’s going to be us with AI”.
That answer made me feel all sorts of ways. Terrified, excited, and deeply curious and inspired to learn a lot more.
This deep dive doesn’t need much of an introduction. AI is the talk of the town. Or better said in the immortalized words of Matagu…
He wasn’t wrong about Hansel, and he wouldn’t be wrong about AI. And that’s largely because of ChatGPT — the natural language chatbot made by OpenAI. We’ll be taking a much closer look at ChatGPT and OpenAI’s strategy here, but in short, it’s the first time AI has had a lot of everyday people experience aha! and oh shit! moments. This is a strong signal that something very important is working at scale, and that we’re on the precipice of an AI revolution.
It’s either going to be great, or it’s going to be terrible. But one thing that’s become very clear to me while researching this — it’s not going to be a fad.
Back in 2018 — AI expert, computer scientist, and former Apple and Microsoft exec, Kai-Fu Lee — said this: “I believe AI is going to change the world more than anything in the history of mankind. More than electricity.” That’s quite the statement…
But, for this to ever be close to happening — AI would need to be widely available and used by the masses. And with the release of ChatGPT, Dall-E and Stable Diffusion in 2022 — we edge closer to exactly that.
In the words of Sam Altman, who amongst many other things founded OpenAI and has been writing and talking about AI for the last 10 years:
I think this is going to be a massive trend, and very large businesses will get built with this as the interface, and more generally [I think] that these very powerful models will be one of the genuine new technological platforms, which we haven’t really had since mobile. And there’s always an explosion of new companies right after, so that’ll be cool.
— Sam Altman, via Greymatters Podcast
That would indeed be cool.
It means new startups will emerge, carving out verticals for themselves and fine tuning their AI to be specialized for certain problems/markets/industries (i.e AI for law, AI for healthcare). To most people, for a long time, AI will likely be this magical thing that’s never fully understood. And it will be the companies that take that magic and make it easy and accessible for people to wield as part of their daily lives that will create a ton of enduring value. For example, a company like Jasper — who bottle the power of AI into a neat Web2 interface, making it approachable and useable for content marketers. In just 2 years, they’re already worth over $1.5b.
In today’s post, we’ll be looking at a company that is laying down essential AI infrastructure (i.e APIs, large language models) to enable all of this. OpenAI, the company Microsoft are looking to acquire a 49% stake in for $10 billion. Or better said, the horse and jockey they want to bring to the next most significant race in tech.
We’ll start with a need-to-know primer on AI, including a quick look at how we got here and an easy to digest overview of the AI market structure. Then, we’ll move into OpenAI’s story and growth strategy.
A ton of research went into this piece given how little I know on this space, and honestly, it has been the most difficult one to write. I feel like this is not me staying in my lane… but, it is what it is…✌️ I’ve learned a lot, and at the minimum, I hope you walk away with a better understanding for the next, inevitable, conversation about AI and ChatGPT you’re roped into. If you do, consider subscribing if this is your first time reading HTG, or sharing this post/newsletter with a friend.
As an aside: This deep dive is not me making a case for or against AI. I think there are some serious risks involved (alignment problem, weapons, the data AI is trained on having bias, job displacement, hiccups like accidental shutdowns of power grids…). Some risks are existential, some less so — but unfortunately humans have a tendency to build things because we can, regardless. The reason I’m writing this is that we all know AI is something right now. It’s here, so we should learn about it.
Let’s begin.
⚠️ If you’re reading this on email, hit this link to read the full thing. ⚠️
A primer on AI — the next revolution
Let’s start off by taking a complicated thing and giving it a simple definition.
What is AI…
Since you’ll sooner catch me eating a cinnamon bagel with lox than use a dictionary definition, I thought I’d kick it off today by crowdsourcing a definition for us, and seeing how close to accurate we get.
To help me with that, I reached out to few people from different industries and asked them, “How would you define AI, and how do you think something like ChatGPT might affect your profession in the future?”. Here’s what I got:
From a senior macro trader
[AI is] any technology or system that assists with simplifying the output that would traditionally require advanced coding/data analysis for any individual - typically using natural language processing, machine learning, or other techniques.
In my industry [macro finance], information/data is abundant but time is arguably the scarcest resource. AI strategies have been a staple in modern financial markets. However, ChatGPT democratizes AI to those looking to automate and speed up simple day to day tasks — succinct news/transcript summaries, write code for backtesting, find patterns across numerous data series. Also, once the data becomes up to date, the software will allow for very simple backtesting strategies, which reduces signal to noise ratio and can generate serious alpha (as long as you’re one of the first users).
From a writer and content marketer
I would define AI as a technology capable of generating unique outputs (responses) based on multiple data points (can even be content) that not only stores historical data but incorporates it into future outputs (AKA it learns).
I imagine that something like ChatGPT will initially automate many pieces of the writing process. I believe that in the near future it will excel at doing 3 out of 4 of the main components in writing content: 1. research, 2. structure, and 3. composition. However, I think editing (the 4th component) will still be human-handled for a while due to its nuances (Google Docs & Microsoft Word can't catch every grammatical nuance) as well as its tone (i.e. you want it to sound human). Additionally, I think it will still require the assistance of a content marketer to guide the prompt (factors like length, topic, main points, etc).
For that reason, I think my job as a content marketer will look very different in the future. There will be a much greater focus on strategy and planning, meanwhile execution will be minimal.
From a filmmaker/producer/photographer (my dad)
AI is something that has been talked about and experimented upon for decades. I understand it as HUMANS giving masses amount of data input into computers, supplying enough information to a system that has been programmed (by people) to rework, reevaluate and calculate millions of possibilities about anything it has been trained or fed and come up with logical options in milli seconds. The AI can assume what would be there but isn’t.
I think the introduction of ChatGPT has its risks long term. As Descartes said, "I think therefore I am". Now we as people may stop doing that. The AI can give so many options and create images and films without the talent of creative people. This will present a whole new look. The individual personal creative inputs, thinking, and errors will be missing and the ART of film making and photography will change.
From a software engineer
AI is ability for a computer to conceptualize and understand an input that isn’t based on a strict set of instructions, and provide a meaningful response.
ChatGPT is just a small subset of AI, and while useful, will provide limited use in its current form. ChatGPT from what I understand was partially trained on coding models and thus explains its ability to write code, however as models improve and PCs get stronger, I believe that an engineers job will change fairly significantly. Even right now, most coders write in a fairly high level language, one that is far away from machine code. I think what will end up happening is engineers will just take one step further away from the machine level, e.g: instead of an engineer writing the exact code, they will utilize AI models to generate specific code to solve specific problems
From a commercial real estate director
My understanding of AI is the ability to harness large volumes of information on the internet at a very rapid rate and develop unique outcomes for particular situations.
The application of AI and programs like ChatGPT will have uses in my industry [commercial real estate] and my be transformative in certain areas, but not all of the industry. In the future I see it helping streamline certain functions (like marketing and content development) and reduce certain traditional roles or expertise while also creating new roles around this technology. Research and market data could also benefit from accessing information provided by AI.
Okay, that was a fun exercise. Now let’s hear from the horses mouth.
💁 Me: Please can you explain what AI is to me like I'm 10 years old. As well as what you are and how you fit into this overall concept of AI. And lastly, can you tell me what you think your purpose is?
🤖 ChatGPT: Sure! AI stands for Artificial Intelligence, which means that it's intelligence created by people, instead of something that happens naturally. Just like how a toy robot can be programmed to move and make noise, AI can be programmed to think and make decisions.
I am a computer program that can understand and respond to human language. I was made by a team of people at OpenAI, who trained me on a lot of text so that I can understand and respond to questions like the one you just asked.
As for why I was made, I think my creators wanted to make a tool that can help people find information and complete tasks more easily. Just like how a hammer can help someone build a house, I can help people find information and answer questions.
💁 Me: Got it. And what do you think some of the biggest misconceptions are about AI are?
🤖 ChatGPT: There are several misconceptions about AI that are widely held:
AI is a single technology: AI is not a single technology, but rather a collection of related technologies that can be applied to various problem domains.
AI will soon become smarter than humans: While AI has made significant progress in recent years, it is still far from surpassing human intelligence in most areas.
AI will take over the world: This is a popular fear, but it's unlikely to happen. AI systems are designed to perform specific tasks and are controlled by humans. They do not have their own goals or desires.
AI is only for big tech companies: AI is not only for big tech companies, but also for small and medium-sized businesses. AI can be used in many industries, such as healthcare, finance, transportation, and retail.
AI is only for the future: AI is not only for the future, it's being used today. AI is already being used in many applications, such as speech recognition, image recognition, and self-driving cars.
AI can replace human jobs: AI can automate some tasks but also can create new jobs in fields such as data analysis, software development, and AI engineering.
It's important to remember that AI is a tool, and its impact will depend on how it is used. The best way to understand AI is to learn about its capabilities and limitations, and to consider the ethical and societal implications of its use.
💁 Me: Thanks for your help today.
Hmm, that was my first conversation with ChatGTP. I’m impressed. 👀 That right there is a great example of how something like ChatGPT is coming for traditional search, and a huge reason Microsoft want them. Answers are often more useful than links.
Anyway, all of ChatGPT’s points there are worth expanding on. But let’s look at point #5, people think AI is only for the future. I think the answer as to why is fairly straightforward…there are just different types of AI. Take my dads answer from earlier: “AI is something that has been talked about and experimented upon for decades.”
He’s right, but it’s also something we’ve already been using for years, everyday. For instance:
Virtual assistants (Siri/Alexa)
Recommendation engines for e-com (Amazon)
Recommendation engines for content (Netflix/Spotify)
Spam filters (Gmail)
Social media algorithms (Instagram/TikTok)
Navigation (Waze)
Fraud detection systems (Chase, Amex)
Voice recognition software (Verizon Call Center)
AI-powered chatbots (Intercom, Drift)
Cybersecurity (Darktrace)
That’s all AI, just not always the way we think about it. So let’s get on the same page with what the different types of AI are before moving into the more meaty stuff…because I too have no idea. We’re in this together folks. 🤘
The different types of AI
AI is categorized by what it’s capable of. Often this is just into three categories (Narrow, Strong, and Super AI). But because this is a deep dive and I want to leave you knowing more than the usual…I bring you 8 types of AI.
Reactive Machines: The simplest type of AI, which can only react to the current situation and can’t form memories or use past experiences (i.e learn) to inform future decisions. e.g: IBM's Deep Blue — the first computer that defeated a chess world champion
Limited Memory: AI that can remember past experiences and leverage them with future decisions. e.g: Self-driving cars, which respond to their environment based on “memories”
Narrow AI: This AI is designed to perform a specific task, such as image recognition, speech recognition, or natural language processing. It’s trained on a large dataset to generate output responses. e.g: ChatGPT, Siri, self-driving cars, recommendation engines, Google maps
Above is all the AI that is currently available to us. A really important point here is that no AI available today creates net new knowledge. In other words, it’s not discovering things and adding to the sum of all human knowledge. It just leverages what we already know, acts as an efficiency tool, and helps us be net more productive. Simply put, ChatGPT won’t give you an answer that isn’t on the public domain of knowledge (the internet), but it will help you find what you need from a snapshot* of the internet much faster. *(Currently ChatGPT has training data/knowledge up until 2021)
Below is the AI that is being worked on that could well bring us to net new knowledge — ranked by stages of progression.
4. Artificial General Intelligence: (AGI) This will be able to understand, learn, and perform any intellectual task that a human can. According to Sam Altman, “AGI is basically the equivalent of a median human that you could hire as a coworker. And then they could do anything that you’d be happy with a remote coworker doing just behind a computer, which includes learning how to go be a doctor, learning how to go be a very competent coder. And I think one of the skills of an AGI is not any particular milestone but the meta skill of learning to figure things out and that it can go decide to get good at whatever you need.”
…i.e ChatGPT ⇒ on steroids ⚡
5. Theory of Mind: This type of AI will be able understand the mental states of other entities, such as humans or other AI, and use that information in this responses and actions.
…i.e therapists ⇒ therAIpist ™
6. Self-Aware: AI that has a sense of self and is able to understand its own mental state. …
i.e it ⇒ he/she/they
7. Strong AI: This would be like AGI + Theory of Mind AI + Self-Aware AI. It would be, for lack of a better term, consciousness.
…i.e me ⇒ a robot 🥲
8. Super AI: Currently only a hypothetical concept. This would be an AI that can perform any task better than a human (or all of humanity combined), would be sentient, has emotions, and could be some seriously scary shit. Think Westworld, Blade Runner, SkyNet. If you asked this dude about Super AI…he might tell you this.
We’re some way away from that…maybe.
If you’re like me, you probably haven’t heard of the The Law of Accelerating Returns
Simply put, it’s compound interest for technological progress.
That means the rate of change in technology exponentially increases. We’ve seen that overall as humanity, but also in each and every single revolution that happened, and with specific technologies inside those revolutions, like computers.
Here’s a tweet from Sam Altman in the days following the release of ChatGPT, saying exactly that:

So, let’s see how we got to where we are today. Or, the flat looking backwards.
A quick history lesson
Here are the highlights of AI’s 83 year history.
1942: Sinking German ships 🪖
During WW2, the Enigma code was broken thanks the Bombe machine designed by Alan Turing. It dramatically sped up the decoding of encrypted messages and allowed the allies to react and strategize much quicker. This was the first application of AI (although the term didn’t exist until 1955), and it that likely shortened the war and saved millions of lives.
We’re off to a good start here.
1948: Playing chess ♟️
Sometimes after helping win a war, you need to make a game for people. So, that’s what Alan Turing did with Turochamp — AKA Turing Chess. It was the trained “program” to play chess.
1950: The imitation game 🤥
Alan Turing published “Computing Machinery and Intelligence” and proposed the idea of “the imitation game” — an experiment to test for machine intelligence. A machine would pass the test if its behavior/responses were indistinguishable from a human. It’s now known as The Turing Test, and no AI has ever passed it.
1957: The first chess-playing computer 🖥️
IBM researcher Alex Bernstein created the first complete chess program to run on an actual computer (i.e not Turing’s wooden box).
1958: Lisp is developed 🧑💻
John McCarthy (the guy who coined the term AI) developed Lisp, the programming language which has become one of most popular languages used in AI research.
1961: The first industrial robot 🦾
Unimate became the first industrial robot. It was a 4,000 pound robotic arm that was used on a General Motors assembly line to streamline metalworking and welding.
1964: The first chatbot 💬
Eliza, the first chatbot, was born. Interestingly, the first use case of an AI chatbot was in psychotherapy. Eliza was trained to give pre-determined responses to the problems users gave it.
1966: The first general-purpose robot 🤖
1966 saw the creation of the first general-purpose mobile robot powered by AI. It’s name was Shakey. Unlike its predecessors, Shakey did not need to be instructed on each individual step of a complex process. Rather, it could analyze commands, break them down, and reason through its actions.
1979: The first self-driving vehicle 🚙
The Stanford Cart became one of the earliest examples of an autonomous vehicle in 1979. It successfully crossed a chair-filled room without human intervention in about five hours.
1993: A warning… 💀
In 1993, Vernor Vinge published “The Coming Technological Singularity”. He predicted that we’ll have the technological means to create superhuman intelligence (Super AI) within thirty years. He also predicted that the human era would end shortly after. 😬
1995: The A.L.I.C.E chatbot 🧑
A.L.I.C.E (Artificial Linguistic Internet Computer Entity), built by Richard Wallace, was released in November 1995. Although inspired by Eliza, there were major tweaks, like it’s integration with NLP (Natural Language Processing), making the conversation feel more natural.
1997: Man vs Machine 🤔
Not quite The Terminator yet, but IBM’s Deep Blue became the first computer chess-playing program to beat a reigning world chess champion, Garry Kasparov.
This left people wondering: how much could machines outsmart humans?
2002: Aah yes, the AI vacuum. 🧹
AI soon absorbed the sponge of cleaning as well. With the introduction of Roomba, cleaning at home became much more efficient.
2006: A pioneering approach to deep learning 🌐
In “Learning Multiple Layers of Representation”, Geoffrey Hinton proposed the ideas that led to “multilayer neural networks that contain top-down connections and training them to generate sensory data rather than to classify it”. Not sure exactly what that means, but it ended up forming the basis of a key approach to deep learning (which is the subset of AI OpenAI use).
2011: AI plays Jeopardy! 📺
IBM’s Watson, a natural language question-answering computer, played Jeopardy! and defeated the reigning champions. The televised game marked AI’s progress and got a lot of people talking.
2011: Hey, Siri 📱
Siri came to the iPhone. This was the first widespread access to a voice assistant powered by AI.
2015: Keep it away from the guns, please. 🤦
Elon Musk, Stephen Hawking and Steve Wozniak were among 3,000 others to sign an open letter requesting a ban on the development and adoption of AI powered weapons for war purposes.
Autonomous weapons have been described as the third revolution in warfare, after gunpowder and nuclear weapons.
This kind of stuff could be terrifying.
2016: A robot becomes a citizen 🇸🇦
Sophia, a humanoid robot that can imitate humans’ facial expressions, language, speech skills, and opinions on pre-defined topics, is given citizenship to Saudi Arabia. What was truly unique about Sophia though was that she (is it a she?) was designed to get smarter over time.
2016: Google’s Deep Mind wins AlphaGo ⚫ ⚪
In March 2016, Google DeepMind's AlphaGo AI program went up against Lee Sedol, one of the world's highest-ranked Go players, in a five-game match. When it was over, Sedol had only won a single match. For decades, researchers had considered Go the Mount Everest of achievements in AI, because Go, which dates back to ancient China, involves a ton of strategy. Unlike chess, which after the first 2 moves has a total of 400 possible next moves, Go has 130,000.
In addition to intelligence, Go requires ingenuity and improvisation. These additional aspects made AlphaGo's achievement even more remarkable. The victory pointed to a near future in which AI would no longer be confined to a narrow series of tasks.
2017: Google creates transformer models 🇸🇦
One of the bigger recent breakthroughs was the emergence of Transformer models, or natural language processing (NLP). Invented at Google, but quickly used by OpenAI to create GPT-1, and most recently GPT-3. This kicked off a bunch of new transformer models being built, like Cohere, A21 Eleuther, as well as for uses in image/voice with the likes of Dall-E, MidJourney, Stable Diffusion, and others.
2018: AI outscores humans 🤓
In 2018, Alibaba developed an AI model that scored better than humans in a Stanford University reading and comprehension test.
2020: AI helps us beat COVID 👩🔬
It typically takes years, if not decades, to develop a new vaccine. One of the reasons we got a COVID vaccine so quickly is because of the AI models that helped researchers analyze vast amounts of data about the virus and identify which compounds were likely to be most effective.
2020: A revolutionary tool for automated conversations 👀
OpenAI unveiled the latest iteration of its Generative Pre-Trained Transformer language modeling algorithms. Kindly abbreviated to GPT-3. This has been the foundation for ChatGPT.
2022: ART-ificial Intelligence 👨🎨
Using Midjourney, an AI program that can turn text descriptions into images, an artist won a notable art competition. This kicked off the debate about the use of AI in art. DanT wrote a great opinion piece on AI’s role in art.
2022: ChatGPT is launched 🚀
November 30, 2022. ChatGPT goes public, and in just 5 days reaches 1 million users. That’s an unprecedented rate. Below is a popular visual that’s made the rounds.
Sure, the definition of a user here is important. Becoming an iPhone user required going to a shop and buying one, vs ChatGPT being a free account made online. But, no matter how you cut the cake, OpenAI distributed ChatGPT extremely fast to the right people — causing awareness and adoption to explode. We’ll get more into that when looking at their GTM motion.
So, that’s a quick recap of AI’s short 83 year history. A little scoop on what came before is always important in getting a sense of where we are, and what might come next.
Now, to have a productive look at OpenAI and the game they’re playing, there’s a need-to-know understanding of the AI market.
Here it is. 🔍
The AI landscape today — the essential overview
Let’s break it up like this:
How the AI market is structured (a 3 layered stack)
What the current market looks like
The AI market structure
For the sake of this deep dive, and a usable-in-conversation understanding, I think the best way to explain how the AI industry is structured is this:
There are three layers.
The first layer is core platforms and infrastructure for AI.
The second (middle) layer is specialized AI models.
The third is the application layer — i.e useable products/services built off L1 and L2
Let’s go a bit deeper.
Layer 1 is these large language models (LLM), and the hosting services for them. It’s the foundational stuff that everybody else is going to get to enjoy. There will probably be just a few of these companies (i.e an oligopoly), with companies like OpenAI. This is analogous to what cloud computing does (AWS, Microsoft Azure, Google Cloud). It’s super expensive to play that game at scale. So thankfully, they’ve taken care of the server problem, enabling builders like us to go and solve specific customer problems.
Layer 2 is the highly tuned, more compact, AI that will be built against the foundational models provided by layer 1 companies (via APIs). This is where there will be a ton of business opportunity on the table. It’s the specialization/verticalization of AI.
Layer 3 is all the commercial applications that will come from layer 1/2. These are the end-user facing tools you and I will actually be using. Examples here are Midjourney, Jasper and Github Copilot.
Here’s an excerpt from a fireside chat between Sam Altman and Reid Hoffman:
I think there will be a small handful of fundamental large models out there that other people build on. But right now what happens is a company makes a large language model (API enabled to build on top of it), and I think there will be a middle layer that becomes really important, where I’m skeptical of all of the startups that are trying to train their own models. I don’t think that’s going to keep going. But what I think will happen is there’ll be a whole new set of startups that take an existing very large model of the future and tune it.
I think there’ll be a lot of access provided to create the model for medicine, or using a computer, or a friend, or whatever. And those companies will create a lot of enduring value because they will have a special version of [the AI]. They won’t have to have created the base model, but they will have created something they can use just for themselves or share with others that has this unique data flywheel going that improves over time.
So I think there will be a lot of value created in that middle layer.
— Sam Altman, founder/CEO of OpenAI
And in a separate conversation between Reid Hoffman and Elad Gil, Reid said he was 100% certain that in the next 5 years there will be “a co-pilot for every profession”, and that he thinks “there will be something [built on AI] for everything”. He added that he thinks that’s a generous estimate.
In other words, not to put words in Reid’s mouth here…but this AI revolution is going to be like the internet revolution. We had this huge wave of startups built off skeuomorphism (i.e “it’s this real world thing, but on the internet!”) that generated tons of online businesses and trillions in value. And now with the progress of AI, it’s going to be, “it’s this internet thing, but with AI”.
That opportunity will either be grabbed by existing companies in the space moving to integrate AI, or new startups rushing in, using this groundwork provided by the likes of OpenAI. i.e We’ll probably see stand alone (de-novo applications built on top of the platforms), as well as tech-enabled incumbents (products where existing companies will “just add AI”)
And it’s in this layer 2 and layer 3 where there is amazing startup opportunity. 🌈
One of the remarkable things about this current technology wave is the speed of innovation across many areas. Future GPT-like language models (GPT-4? GPT-N?) should increase the power, fidelity, and reach of natural language across consumer and B2B in deep ways and potential change everything from human interactions (dialogue based interactions?) to white collar work (co-pilot for anything that touches text, by vertical). In parallel, advances in image generation, speech to text, text to speech, music, video, and other areas are happening. One can imagine 4-5 clear business use cases from image-gen, from better versions of various design tools to storyboarding for movie making. Which of these uses cases are won by startups versus incumbents remains to be seen but one can guess for a subset based on the strength or nimbleness of existing incumbents.
This time, the technology seems dramatically stronger, which means it is easier to create 10X better products to overcome incumbent advantages. The "why now" may simply be a technology sea change.
The pivot point for whether now is the moment where AI takes off for startups depends on whether GPT-4 (or some other API platform) is dramatically more performant than GPT-3/3.5. GPT-3 seems to be useful but not "breakthrough" useful to the point where large numbers of startups are building big businesses on it yet. This could also just mean not enough time has passed since it launched recently. However, a 5-10X better model then GPT-3 should create a whole new startup ecosystem while also augmenting incumbent products.
Well… according to multiple sources (although nothing I could find confirmed by OpenAI) — GPT-4 could be in the region of 500x better. 🤯 As a reference, GPT-3 was ~100x better than GPT-2 (175b parameters vs 1.5b). Don’t ask me to explain parameters, just know it’s a key measure in the AI performance.
So if Elad, Sam, and Reid are right…the wave is indeed coming and the AI sector (currently valued at $120b) is going to explode.
And when it does, the players who enter the game here will still be playing by the old school rules. It will be the startups/companies that have superior distribution, network effects, are first to get to scale, can aggregate customers, build and position a brand well, aggregate talent, and aggregate the capital fastest, that will win.
The thing is…it’s already happening, especially with generative AI (i.e AI that creates content based on a prompt).
Market map of generative AI
We’re starting to see the very early stages of a tech stack emerge in generative artificial intelligence. Hundreds of new startups are rushing into the market to develop foundation models, build AI-native apps, and stand up infrastructure & tooling.
Many hot technology trends get over-hyped far before the market catches up. But the generative AI boom has been accompanied by real gains in real markets, and real traction from real companies. Models like Stable Diffusion and ChatGPT are setting historical records for user growth, and several applications have reached $100 million of annualized revenue less than a year after launch.
There is enough early data to suggest massive transformation is taking place. What we don’t know, and what has now become the critical question, is: Where in this market will value accrue?
Infrastructure vendors are likely the biggest winners in this market so far, capturing the majority of dollars flowing through the stack. Application companies are growing topline revenues very quickly but often struggle with retention, product differentiation, and gross margins. And most model providers, though responsible for the very existence of this market, haven’t yet achieved large commercial scale.
To visualize the ecosystem right now, check out this awesome map put together by Base10.
That’s a good chunk of companies, but…
In prior technology cycles, the conventional wisdom was that to build a large, independent company, you must own the end-customer — whether that meant individual consumers or B2B buyers. It’s tempting to believe that the biggest companies in generative AI will also be end-user applications. So far, it’s not clear that’s the case.
To be sure, the growth of generative AI applications has been staggering, propelled by sheer novelty and a plethora of use cases. In fact, we’re aware of at least three product categories that have already exceeded $100 million of annualized revenue: image generation, copywriting, and code writing.
However, growth alone is not enough to build durable software companies. Critically, growth must be profitable — in the sense that users and customers, once they sign up, generate profits (high gross margins) and stick around for a long time (high retention). In the absence of strong technical differentiation, B2B and B2C apps drive long-term customer value through network effects, holding onto data, or building increasingly complex workflows.
In generative AI, those assumptions don’t necessarily hold true. Across app companies we’ve spoken with, there’s a wide range of gross margins — as high as 90% in a few cases but more often as low as 50-60%, driven largely by the cost of model inference. Top-of-funnel growth has been amazing, but it’s unclear if current customer acquisition strategies will be scalable — we’re already seeing paid acquisition efficacy and retention start to tail off. Many apps are also relatively undifferentiated, since they rely on similar underlying AI models and haven’t discovered obvious network effects, or data/workflows, that are hard for competitors to duplicate.
So, it’s not yet obvious that selling end-user apps is the only, or even the best, path to building a sustainable generative AI business. Margins should improve as competition and efficiency in language models increases (more on this below). Retention should increase as AI tourists leave the market. And there’s a strong argument to be made that vertically integrated apps have an advantage in driving differentiation. But there’s a lot still to prove out.
— a16z
Now that we know what AI is and have a decent sense of the market, we can have a look at one of the most important companies in it.👇
Open sesame — OpenAI’s beginning
A dinner and a billion dollars
It all started in August 2015, around a dinner table in Menlo Park, California.
It was a regularly scheduled evening with Sam Altman, Elon Musk, Greg Brockman, and Ilya Sutskever. One where they came together to talk about big ideas.
During this particular meal, the conversation quickly turned to AI.
We'd each come to the dinner with our own ideas. [At this one] we talked about the state of the field, how far off human-level AI seemed to be, what you might need to get there, and the like. The conversation centered around what kind of organization could best work to ensure that AI was beneficial.
It was clear that such an organization needed to be a non-profit, without any competing incentives to dilute its mission. It also needed to be at the cutting edge of research (per the Alan Kay quote, “the best way to predict the future is to invent it”). And to do that, it would need the best AI researchers in the world
So the question became: would it be possible to create from scratch a lab with the best AI researchers? Our conclusion: not obviously impossible.
"Elon and Sam had a crisp vision of building safe AI in a project dedicated to benefiting humanity," Brockman recalls. They then floated an idea that went against the status quo of AI development at big tech incumbents. Instead of intensively training algorithms behind closed doors, build AI and share its benefits as widely and as evenly as possible.
Shortly after the dinner, on December 11 2015, OpenAI was founded as a non-profit by the four dinner guests to “develop safe and friendly AI systems”. They quickly raised $1 billion from Musk, Altman, and other prominent Silicon Valley characters, like Peter Thiel and Reid Hoffman.
💡 Takeaway: Network with like-minded people (or better yet, form a brain trust) and make time to discuss hard problems and interesting ideas. You never know what might come from it.
The build up to ChatGPT
With their growing team of researches and engineers, OpenAI has been running lots of experiments. They’ve developed AI that can beat humans at video games (OpenAI Five), built an environment for developing and testing reinforced learning agents (OpenAI Gym), and incredibly, they launched a platform for developing and testing an AI against the world's supply of websites, video games, and other applications. It’s called OpenAI Universe.
[The goal with Universe] is to develop a single AI agent that can flexibly apply its past experience on Universe environments to quickly master unfamiliar, difficult environments, which would be a major step towards general intelligence.
In other words…Universe has unleashed an AI that is busy learning to master whatever game or task it gets access to. Every time it wins, it learns, and it goes into the next task with all those memories — exponentially getting better. Wild.
I doubt you’ve ever heard about any of those three projects. But if you’re an engineer, you may well have heard of the next one. I think this AI in the next few years is going to seriously change the game for non-technical founders.
💡 Takeaway: Get your product out there as fast as possible. Experiment, learn, and don’t worry about being in stealth mode. Feedback and field data is far more important.
Codex — creating code
Imagine being able to type out your idea in natural language and have it turned into code.
Well, with OpenAI’s Codex…you can easily turn English instructions into over a dozen popular coding languages, including Python.
OpenAI Codex empowers computers to better understand people’s intent, which can empower everyone to do more with computers.
Once a programmer knows what to build, the act of writing code can be thought of as (1) breaking a problem down into simpler problems, and (2) mapping those simple problems to existing code (libraries, APIs, or functions) that already exist. The latter activity is probably the least fun part of programming (and the highest barrier to entry), and it’s where OpenAI Codex excels most.
OpenAI Codex is a general-purpose programming model, meaning that it can be applied to essentially any programming task (though results may vary). We’ve successfully used it for transpilation, explaining code, and refactoring code. But we know we’ve only scratched the surface of what can be done.
Just watch this (even if just the first minute)…holy smokes. 🤯
When I was a founder, I had wonderful/wishful dreams of being able to actually sit down at a computer and build out my ideas myself. This is going to make that possible for people, and it’s bloody magical.
This is the single most mind-blowing application of machine learning I’ve ever seen.
— Mike Krieger, Co-founder of Instagram
Codex is the AI model that powers GitHub Copilot and is already in the field being used by devs at major companies.

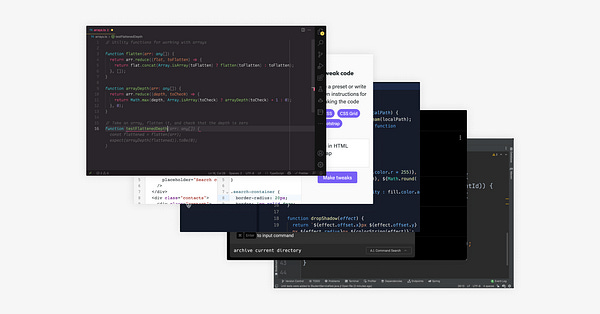
The next project is one you may well have heard of.
DALL-E — creating images
DALL-E is like Codex for images. As an example:
The art created is all about your prompt…and your prompt can literally be anything. You can play around with your concept, add descriptors, change the style — and the AI will create you a bunch of images for you to do whatever you want with, including sell them.
DALL·E 2 has learned the relationship between images and the text used to describe them. It uses a process called “diffusion,” which starts with a pattern of random dots and gradually alters that pattern towards an image when it recognizes specific aspects of that image.
— via OpenAI
And we’re not just talking pixel astronauts…
The latest version of DALL-E is making images like that. Incredibly realistic
The possibilities from here are not too hard to image.
If we’re creating images…we’re just one little step away from videos.
Remember…a video is really just lots of images (frames) sequenced together very quickly. And if AI is able to create images like that extremely quickly…why not video?
The applications of that will be huge. Think generative content in video games (characters, entire worlds), simplifying production of entertainment content, ads…the list goes on.
If I were a stock library (i.e Shutterstock, Getty)…I’d be taking this extremely seriously and looking at how to make this a core part of my business.
Now, I deliberately spoke about Codex and DALL-E first to show you some pretty amazing use cases of the first two products built on OpenAI’s foundational NLP (natural language processing model). Both of those tools are built on modified versions of GPT-3.
Okay, so wtf is all this GPT stuff?
GPT-1, 2, 3
GPT-3, or the third-gen Generative Pre-trained Transformer, is a neural network machine…
Um…
Simply put, it's an AI that is better at creating content based on text inputs than anything that has come before it.
All it needs is a natural language prompt from you and me (input), and based on a deep learning language prediction model that has been pre-trained on a snapshot of the world’s data (i.e a crawled internet), it can then output whatever text response back to us that it thinks is the most useful knowledge. But like I said earlier…it doesn’t produce any net new knowledge. It can tell you something you don’t know, but it can’t tell you something nobody knows.
What can GPT-3 do?
Long before we got out hands on ChatGPT, OpenAI released the beta of GPT-3 on June 11, 2020 and made their API available by request. They wanted to "explore the strengths and limits" of this new technology, and it’s how companies like Jasper got ahead of the market demand.
The latest iteration of this LLM (large language model) can create anything that has a language structure — meaning it can answer questions, write essays, summarize online articles/books, write long texts, translate languages, take memos, and like we saw with Codex, write computer code.
It’s also a base model (e.g for Codex, DALL-E), that can be modified. Like for design. Check out this demo (~1:30m) of a designer creating an Instagram app in Figma by just prompting their idea.
Shortly after the release of GPT-3, Microsoft (who invested $1b back in 2019) announced that they had licensed "exclusive" use of GPT-3. Others would still be able to use the public API to receive output, but only Microsoft has access to GPT-3's underlying model.
The scope of commercial and creative potential that can be unlocked through the GPT-3 model is profound, with genuinely novel capabilities – most of which we haven't even imagined yet.
Roughly a year later, on November 18, 2021, OpenAI announced that enough safeguards had been implemented that access to its API would be unrestricted. And on January 27, 2022, they announced that their newest GPT-3 language models, collectively referred to as InstructGPT, would now be the default language model used on their API. They said it could produce content that was better aligned to user intentions by following prompts better, generating fewer made-up facts, and producing somewhat less toxic content.
They iterated and improved the model, and on November 30, 2022, they began referring to the model as the GPT-3.5 series and released ChatGPT to the public in an elegant experience akin to searching on Google.
And to give you a sense of the rapid progress made on OpenAI’s GPT product since GPT-1 in 2018…
They say a picture says a thousand words. And really, that’s all you need to know. GPT-3 is that much better than it’s predecessors (100x). Plus, this was all in just 2 years.
Hmm. What about GPT-4?
Like I mentioned earlier, it could be in the region of 500x better — and it could coming this year.
This is serious accelerating returns. It would be the equivalent of a 1 year old baby at their next birthday waking up having lived, learned, and built experiences on about 7 full life times. 🙃
Sam Altman has downplayed/denied this scale, saying people’s expectations are too high and it may not even come out this year. But, even if we got a fraction of that (like Elad suggested)…it would be a massive difference.
Okay, so OpenAI has run a bunch of experiments, launched GPT-3, and has three core products out there; Codex, DALL-E, and ChatGPT.
Why? And what’s their game plan in this rapidly growing industry?
OpenAI’s strategy
OpenAI is already valued at $29bn, but honestly, this is nothing compared to the potential in front of them. They are getting themself into a really great position to earn a significant portion of all NLP category revenues as more startups are built in L2 and L3 — even more so if their integration into Microsoft’s product portfolio goes smoothly.
Let’s start off by looking at the big picture. We’ll then get into the value of ChatGPT in this macro strategy (hint, it’s a wedge), and we’ll round out with a look at the impact of this Microsoft deal.
The long-term play
OpenAI are building an open source platform (layer 1) that will enable this entire new wave of AI startups in layer 2 and layer 3 to exist.
Like Epic Games’ strategy of growing their business by focusing on game creators and growing the entire gaming ecosystem — this is what OpenAI are doing. As their name states fairly clearly…they are opening up AI to entrepreneurs and builders.
In other words, their strategy is:
Build a deep language model (GPT-n)
Demonstrate its capabilities/power and establish platform reputation
Make it easily accessible via APIs
Become the foundation for layer 2 and layer 3 companies
Lock customers in (network effects) and build data moats
Capture a % of all value created across the AI landscape on top of their platform (API usage)
Keep investing in the core model, making it more powerful and accessible (technical moat)
This isn’t novel, but it is brilliant.
The two most recent companies we’ve looked at on How They Grow just so happen to be doing exactly this:
Stripe: Payments infrastructure platform. Toll-booth for payments.
Epic Games: Game engine platform. Toll-booth for video games/entertainment.
And both of those companies, as part of their core strategy, focus on seeding new companies/founders/builders by investing in them and backing them to grow into players that create long-term value and grow the total addressable market (TAM) of their industry. For instance, Epic Games’ publishing division that funds new creators.
OpenAI is taking one from that playbook, too.
Funding their own customers and seeding the wave
Just before the release of ChatGPT, OpenAI said they would give 10 AI startups $1M each and early access to their systems. It’s called OpenAI Startup Fund.
According to their super minimal landing page, this is how they describe it:
The OpenAI Startup Fund is investing $100 million to help AI companies have a profound, positive impact on the world. We’re looking to partner with a small number of early-stage startups in fields where artificial intelligence can have a transformative effect—like health care, climate change, and education—and where AI tools can empower people by helping them be more productive.
And their mandate for this first fund helps us understand who OpenAI are targeting as part of their GTM.
Engineers, designers, researchers, and product builders … from all backgrounds, disciplines, and experience levels are encouraged to apply, and prior experience working with AI systems is not required.
The move here is clear: find the people we want building on our GPT model, give them the resources to be successful while simultanesouly building our brand in the industry, and hope they grow up to be billion dollar companies — benefiting both our fund and platform revenue.
But what about defensibility? Well…
Platforms (always) win.
In our last 5-Bit Friday, I summarized a great essay from the Harvard Business Review: Pipelines, Platforms, and the New Rules of Strategy .
If you missed it, it’s worth a catchup, but in short — pipeline businesses are like Activision (make your own game and sell it), platform businesses are like Epic Games (make a game builder and allow others to sell their games). And platforms always win.
Of course there are going to be other companies that will go and make their own language models. Same with Stripe — some companies want their own proprietary payments system. In the gaming world, companies like Activision want their own engines for games like Call of Duty vs using Epic’s engine.
And that’s totally fine, because for the majority of the market it doesn’t make sense to spend all that time and money building your own proprietary model when you could be focusing on the problem you’re trying to solve. Just tap into the existing model, tune it for your needs, and you’re off to the races.
Okay, but what about competition from other foundational L1 companies?
There are going to be other companies competing at the L1 level of the stack. For example, Google’s DeepMind.
But like we spoke about earlier, there’s a ton of value on the table in an oligopoly. Just look at telecoms (Verizon, AT&T) and cloud computing (AWS, Azure, G-Cloud).
There’s nothing OpenAI can do to stop that from happening, but it likely won’t expand beyond a few players that end up holding huge pieces of the market. The barriers to entry for startups at that point will just be so high — with huge upfront costs, and then the challenge of taking away customers from the likes of OpenAI. And you can imagine the lock-in effect/switching cost of changing the foundation you built your company on top of.
But for the companies that do provide these big models, including OpenAI, they will face some hard questions:
Commoditization. There’s a common belief that AI models will converge in performance over time. Talking to app developers, it’s clear that hasn’t happened yet, with strong leaders in both text and image models. Their advantages are based not on unique model architectures, but on high capital requirements, proprietary product interaction data, and scarce AI talent. Will this serve as a durable advantage?
Graduation risk. Relying on model providers is a great way for app companies to get started, and even to grow their businesses. But there’s incentive for them to build and/or host their own models once they reach scale. And many model providers have highly skewed customer distributions, with a few apps representing the majority of revenue. What happens if/when these customers switch to in-house AI development?
Is money important? The promise of generative AI is so great — and also potentially so harmful — that many model providers have organized as public benefit corporations (B corps), issued capped profit shares, or otherwise incorporated the public good explicitly into their mission. This has not at all hindered their fundraising efforts. But there’s a reasonable discussion to have around whether most model providers actually want to capture value, and if they should.
— a16z
GPT sits at the heart of OpenAI’s business and growth strategy.
But it’s ChatGPT that we’ve all been talking about.
So let’s zoom in.
OpenAI’s GTM strategy with ChatGPT
ChatGPT has grown faster than any other app in history. Nothing else has reached 1 million users as fast, and they are still surging on.
This happened so recently and there’s no clear cut answer or playbook they followed, but here are some of the things OpenAI did extremely well.
A wedge, and a conversation
GPT is the core product. ChatGPT is just the wedge to get people using it.
Once again, looking at Epic Games as a comparison: Epic built Unreal Engine (the platform), but strategically built their video game Unreal on top of it, releasing both at the same time. The game was a hit, getting them widespread attention and demonstrating the power of the engine they built. Creators flocked, and today they have one of the two biggest game engines in the world.
Sound familiar? 🤷♂️
In my view, one of the most brilliant things that OpenAI has done is starting a conversation about AI. They just so happened to do that by allowing everyone else to start conversations with AI. Let’s be real, before ChatGPT came out, AI wasn’t all over out LinkedIn and there wasn’t a mountain of posts, podcasts, and chatter about this new future.
OpenAI opened pandoras box to us — and we all saw the magic. They did this by making their research with GPT available to non-experts (that’s you and me ✌️) in a dead simple UI and allowing us to start playing with it. This is what ignited the conversation about AI outside of people in AI.
And they knew they were releasing magic, so they used one-to-many broadcasts as a fuel to drive people to it. Big tweets, notable press, and a truly novel thing worth talking about took them to 1M users in 5 days.
Here are 3 tweets that were conversation catalysts. There were many more.
How to get press is an interesting topic but outside of our scope here. If you’re curious, here’s a good starting point. But one thing you need to have that ChatGPT nailed, is a magic moment that’s easy to find. 🪄
Easy magic.
A frictionless UI/UX means nothing stops the user from getting what they’re looking for.
Searching on Google is the best example of a frictionless experience. It just works with no user effort. You just type/speak and you get what you came for. And while we’re desensitized to the magic that is bottled in Google Search’s simple UI, we certainly were not when we tried ChatGPT.
We asked, and everything changed.
Their research and access to the GPT model wasn’t buried behind confusing technical steps or long onboarding — it was just there for us to play with. We just needed to give an email and password, which in today’s world, is the equivalent to frictionless.
And that’s a really important lesson for product/growth folks — get rid of unnecessary friction and get users to the core value point as fast as you can, and as often as you can.
*As an aside: the only friction around ChatGPT is getting through. They’re often at capacity.
Let’s look at the next thing OpenAI have done, where the work here started long before the release of ChatGPT.
Building a brand around people’s biggest concerns
Safety, trust, responsibility, governance.
Those words are airy, but they truly have been core part of OpenAI’s charter and brand.
And they have to be, because AI without that will be a total shit show.
OpenAI’s research has been widely recognized in the field, and they’ve done a great job at establishing themselves as a leader in the development of AI technology and a responsible “guardian of this revolution”. And the experiments/projects we looked at earlier were important tools in helping build this reputation.
They’ve also been widely recognized for their contributions to open-source software and actively engaging with the research community (including partnering with other organizations/researches) to promote transparency and collaboration.
Building in a closed environment and just releasing something like ChatGPT would have been a huge mistake. It would have drawn far more concern and distrustful eyes. But by building alongside experts and proving they are executing against their mission of those “airy” terms — that became their brand.
And now that we know ChatGPT is a wedge for GPT, let’s bring this together with a quick Canva creation. 👨🎨
Looking at the bottom left — let’s go deeper on growth via partnerships, and how this deal gives OpenAI a key asset — distribution.
OpenAI x Microsoft — the strategic value
I mentioned earlier that Microsoft invested $1 billion back in 2019 into OpenAI. And now, they have extended their partership with OpenAI are are looking to get a much bigger stake in the business.
In the 9th series of 5-Bit Friday’s, I wrote the following:
On Wednesday, Peter Yang (Product Lead at Roblox), shared this powerful image on LinkedIn.
Takeaway: Microsoft could well be the best positioned company in big tech for the next decade.
Why?
They have hugely diversified revenue streams
Their cloud product (Azure) is the clear #2 in the cloud market
And, significantly, they could be getting massive exposure to AI and the next revolution
And I want to expand on that last point, because it’s huge.
In short, Microsoft are looking to acquire 49% (worth $10b) of OpenAI. OpenAI is the company behind ChatGPT, and according to the terms outlined in Reuters — this could be a sweet deal.
Microsoft would receive 75% of OpenAI’s income until it has recovered its initial investment. Once they hit that threshold, they would have a 49% stake in OpenAI, with other investors taking another 49% and OpenAI's nonprofit parent getting 2%.
So, why would they be doing this, and what could the strategic value be?
First, ChatGPT is far beyond answering questions or being a chat bot. It’s an example of a neural network that is actually working, and what comes next here is both unknown and likely massive.
And Microsoft wants a piece of this for a few reasons:
ChatGPT threatens Google’s search engine. Asking Google a question brings you a list of answers, all from different sources. But, ask ChatGPT, and it brings you one answer based on info across sources. And as Microsoft look to gain market share here by beefing up Bing, this is an important weapon.
Microsoft will most likely deploy OpenAI’s Chatbot and ChatGPT across all their office products. With 1.4 billions users across the world, this would be extremely valuable to OpenAI, as well as solve problems for their office users — leveling up the value of their tools which account for 24% of their business.
OpenAI runs on Microsofts cloud — giving them a great platform for integration and collaboration.
And lastly and probably most importantly, in the words of Yesha Sivan of i8 Ventures:
Microsoft is not willing, again, to lose the next revolution. If you look at the last 20 years, they are very good at losing revolutions. The revolution of the internet they lost, of mobile they lost, etc.
You see it in the metaverse with their $79b investment. They are not willing to lose.
I’m very excited to see how this plays out.
— via 5-Bit Friday’s (#9): Weekly snacks from the startup/tech universe
And since I wrote that on Jan 13th, things have progressed. 🤝
Microsoft said on Monday [01/23] that it was making a “multiyear, multibillion-dollar” investment in OpenAI, the San Francisco artificial intelligence lab behind the experimental online chatbot ChatGPT.
The companies did not disclose the specific financial terms of the deal, but a person familiar with the matter said Microsoft would invest $10 billion in OpenAI.
Microsoft had already invested more than $3 billion in OpenAI, and the new deal is a clear indication of the importance of OpenAI’s technology to the future of Microsoft and its competition with other big tech companies like Google, Meta and Apple.
With Microsoft’s deep pockets and OpenAI’s cutting-edge artificial intelligence, the companies hope to remain at the forefront of generative artificial intelligence — technologies that can generate text, images and other media in response to short prompts. After its surprise release at the end of November, ChatGPT — a chatbot that answers questions in clear, well-punctuated prose — became the symbol of a new and more powerful wave of A.I.
So, let’s expand a bit more on the why behind this deal. And to do that, let’s go back to the question: Where in this new market will value accrue?
We spoke about 3 layers in this stack: (1) AI platforms/Infrastructure, (2) specialized AI, and (3) end-user applications.
But, there’s actually a fourth layer. And now that we’re talking about Microsoft, let’s introduce this deeply important piece of the stack.
It’s the infrastructure for AI-specific infrastructure.
Companies like OpenAI are building these massive models, but just like every other software company in the world — they need computing power. And for all the companies that are building in L1 (like OpenAI) — they’re using hardware (i.e GPUs by Nvidia) and cloud computing services (i.e Azure) to exist. As will all the companies in L2 and L3. In other words…nearly everything in generative AI passes through here.
Let’s call this Layer 0 — it’s where the infrastructure vendors that run training and workloads for generative AI models live. Think of building a house…we all know how essential cement is to the foundation, but we don’t really think too much of the lime producers supplying the cement companies with that essential ingredient to make it.
These layer 0 infrastructure vendors will touch everything.
For the first time in a very long time, progress on the most disruptive computing technology is massively compute bound.
As a result, a lot of the money in the generative AI market ultimately flows through to infrastructure companies. To put some very rough numbers around it: We estimate that, on average, app companies spend around 20-40% of revenue on inference and per-customer fine-tuning. This is typically paid either directly to cloud providers for compute instances or to third-party model providers — who, in turn, spend about half their revenue on cloud infrastructure. So, it’s reasonable to guess that 10-20% of total revenue in generative AI today goes to cloud providers.
On top of this, startups training their own models have raised billions of dollars in venture capital — the majority of which (up to 80-90% in early rounds) is typically also spent with the cloud providers. Many public tech companies spend hundreds of millions per year on model training, either with external cloud providers or directly with hardware manufacturers.
This is what we’d call, in technical terms, “a lot of money” — especially for a nascent market.
With that in mind, and knowing 31% of Microsoft’s business is cloud computing, we can see the benefits that could come around OpenAI existing exclusively inside their Azure ecosystem. And in their own words…
Supercomputing at scale – Microsoft will increase our investments in the development and deployment of specialized supercomputing systems to accelerate OpenAI’s groundbreaking independent AI research. We will also continue to build out Azure’s leading AI infrastructure to help customers build and deploy their AI applications on a global scale.
New AI-powered experiences – Microsoft will deploy OpenAI’s models across our consumer and enterprise products and introduce new categories of digital experiences built on OpenAI’s technology. This includes Microsoft’s Azure OpenAI Service, which empowers developers to build cutting-edge AI applications through direct access to OpenAI models backed by Azure’s trusted, enterprise-grade capabilities and AI-optimized infrastructure and tools.
Exclusive cloud provider – As OpenAI’s exclusive cloud provider, Azure will power all OpenAI workloads across research, products and API services.
— Microsoft and OpenAI extend partnership, via Microsoft Blog
And for all the reasons Microsoft want OpenAI…we can see the same benefits for Google and their OpenAI challenger, DeepMind. The classic rivalry between the two has Google concerned.
Google appears to be renewing its focus on AI after issuing a "code red" over the rise of ChatGPT in December. The New York Times reported that Google had been shaken out of its routine, following the launch of OpenAI's chatbot.
The launch of ChatGPT has sparked concerns about the future of traditional search engines.
What’s more…even Larry Page and Sergey Brin are back in the war room. “The re-engagement of Google’s founders, at the invitation of the company’s current chief executive, Sundar Pichai, emphasized the urgency felt among many Google executives about artificial intelligence and that chatbot, ChatGPT”.
So outside of the tremendous value that’s on it’s way in with this new AI market — two massive existing markets are at stakes here. Search ($167b) and Cloud Computing ($426b).
The strategic importance of acquiring OpenAI is clear for Microsoft, but what about the value for OpenAI? How will it help them grow?
Resources + distribution
For starters, OpenAI doesn’t really make much money right now. They forecast $200b in revenue this year, and just as one expense, it currently costs them about $100k a day to operate ChatGPT.
So resources are the obvious one. They need to fund operations as well as growth as they build out GPT-4.
The second, and most important reason, is distribution. Microsoft have deep enterprise relationships and a huge volume of companies built on their Azure network. OpenAI don’t.
And we can already see Microsoft integrating OpenAI into their Azure platform. This integration (1) enables Azure to become the go-to enterprise platform for the development of custom AI models, and (2) opens up a huge pool of customers to OpenAI.
Even calling it Azure OpenAI — cheeky.
We debuted Azure OpenAI Service in November 2021 to enable customers to tap into the power of large-scale generative AI models with the enterprise promises customers have come to expect from our Azure cloud and computing infrastructure—security, reliability, compliance, data privacy, and built-in Responsible AI capabilities.
Since then, one of the most exciting things we’ve seen is the breadth of use cases Azure OpenAI Service has enabled our customers—from generating content that helps better match shoppers with the right purchases to summarizing customer service tickets, freeing up time for employees to focus on more critical tasks.
Customers of all sizes across industries are using Azure OpenAI Service to do more with less, improve experiences for end-users, and streamline operational efficiencies internally. From startups like Moveworks to multinational corporations like KPMG, organizations small and large are applying the capabilities of Azure OpenAI Service to advanced use cases such as customer support, customization, and gaining insights from data using search, data extraction, and classification.
via Microsoft Blog
Just to illustrate scale here incase you didn’t believe Microsoft had a lot of customers. Azure has penetration with ~ 95% of Fortune 500 companies, has over 145M daily active users on Microsoft Teams, and over 250K companies using Microsoft Dynamics 365 and Microsoft Power Platform, according to Microsoft.
That’s a lot of B2B/enterprise customers that OpenAI doesn’t have to spend to much time worrying about acquiring.
And going back to OpenAI’s big picture strategy:
[…initial steps]
Lock customers in (network effects) and build data moats
Capture a % of all value created across the AI landscape on top of their platform (API usage)
Keep investing in the core model, making it more powerful and accessible (technical moat)
Obviously, this distribution accelerates that for OpenAI.
One more time…
And that closes us out for our deep dive on OpenAI. I’ll wrap up by saying that I'm of the strong opinion that we should progress very mindfully here. That being said, AI is coming, and I don’t want to be an ostrich and stick my head in the sand. So, the alternative is to be excited and open about AI’s future, and pray to the pope it only help us. 🙏
For the most important quote in today’s post…
Right you are, Uncle Ben.
Until next time.
— Jaryd ✌️
If you enjoyed this post, consider sharing it with someone else!
Excellent read. Shared on my LinkedIn.
Just stumbled on this. Wow! It was an interesting and well engaging read Jaryd. Thanks